Hammersley
Hammersley sampling belongs to the category of quasi-Monte Carlo methods. This technique uses a quasi-random number generator, based on the Hammersley points, to uniformly sample a unit hypercube.
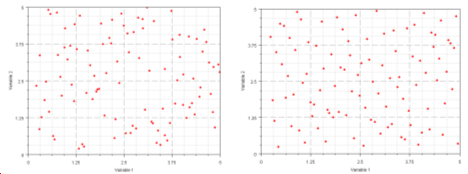
Figure 1. . Latin HyperCube (left) and Hammersley (right) for 100 runs.
Usability Characteristics
- An efficient sampling technique that provides reliable estimates of output descriptive statistics using fewer samples than random sampling. For example, for the same number of runs, a Hammersley sample will be closer to the theoretical mean than a truly random sample.
- Provides good, uniform properties on a k-dimensional hypercube. This is an advantage over Latin HyperCube sampling, which provides good uniform properties of each dimension individually.
- To get a good quality fitting function, a minimum number of runs should be evaluated. (N+1)(N+2)/2 runs are needed to fit a second order polynomial, assuming that most output responses are close to a second order polynomial within the commonly used input variable ranges of -+10%. An additional number of runs equal to 10% is recommended to provide redundancy, which results in more reliable post-processing. As a result, this equation is recommend to calculate the number of runs needed or a minimum of 1.1*(N+1)(N+2)/2 runs.
- Any data in the inclusion matrix is combined with the run data for post-processing. Any run matrix point which is already part of the inclusion data will not be rerun.
Settings
Parameter | Default | Range | Description |
---|---|---|---|
Number of Runs | > 0 integer | Number of new designs to be evaluated. | |
Use Inclusion Matrix | Off | Off or On | Concatenation without duplication between the inclusion and the generated run matrix. |